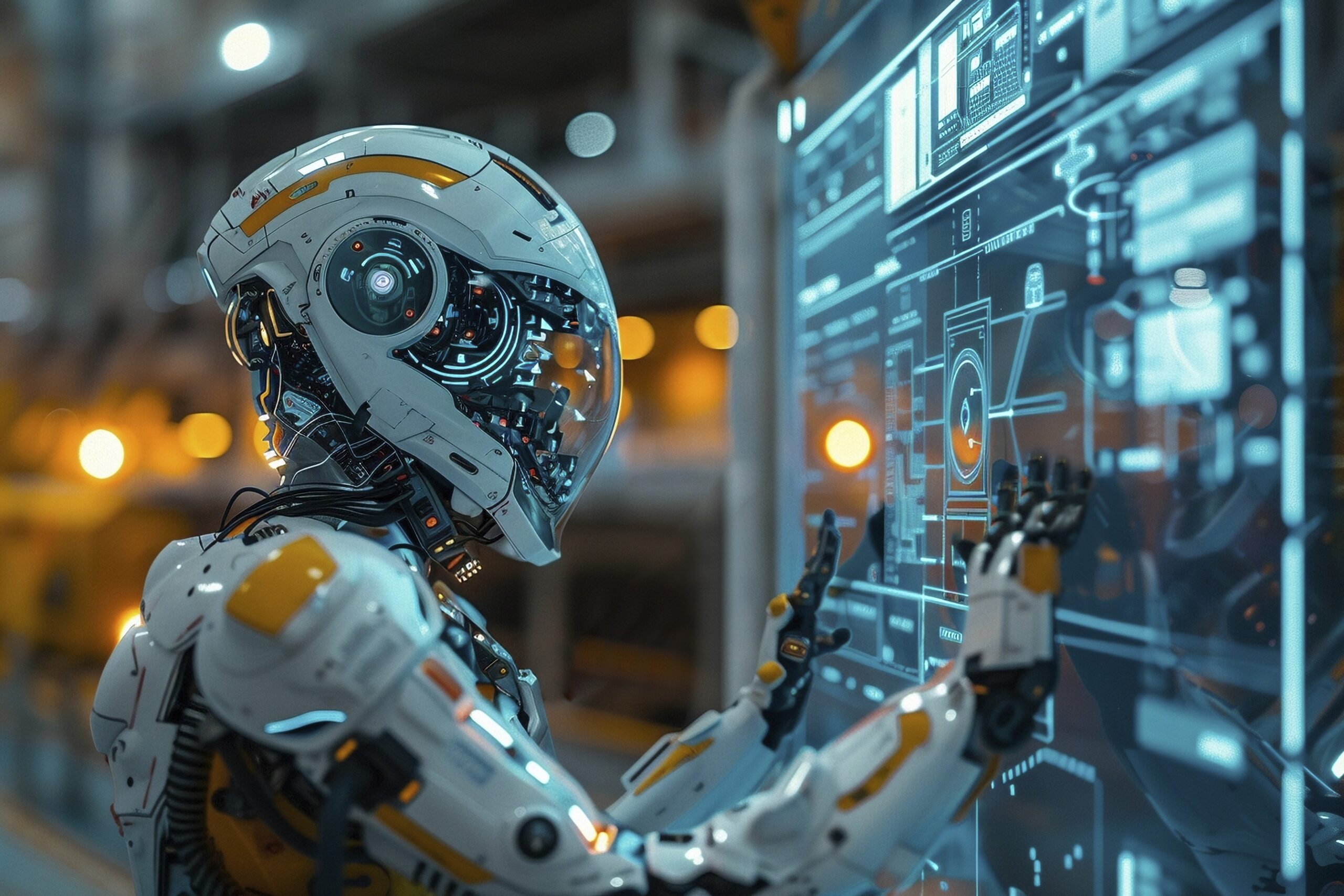
An average tech enthusiast or a layman that is unfamiliar with the intricacies of the tech world would often confuse the terms Artificial Intelligence (AI) and Machine Learning (ML). This is largely because many tech professionals often use these terms interchangeably, despite the fact that they don’t mean the same thing. Additionally, there are others do not fully understand the distinct functions of Machine Learning and Artificial Intelligence, leading to an underappreciation of these two technological concepts. This has birthed several questions, which we intend to explore in this post.
Common Questions About AI and ML
Are AI and ML the same thing? or Are they similar? Can we have AI without ML, and vice versa? If they are different, how are they related? These are common questions asked by businesses, professionals, and tech enthusiasts alike, as they attempt to unravel the mysteries behind these technologies.
In the first part of this post, we will be diving into the similarities between Machine Learning and Artificial Intelligence. We will then proceed to explore their unique features, thereby helping to clarify their individual contributions to the tech ecosystem.
To fully understand the similarities between these two major driving forces in the tech world, it’s crucial to first take a quick look at what each term means.
What is Machine Learning?
Machine Learning is a subset of AI that focuses on the development of algorithms that allow computers to learn from data and make decisions based on that data. ML algorithms can improve their performance over time through experience, making them unlike traditional programming. This process is called “training.”
What is Artificial Intelligence?
Artificial Intelligence is a broad field in computer science that seeks to create machines capable of mimicking human intelligence. At its core, AI involves building systems that can perform tasks that typically require human cognition, problem-solving, reasoning, understanding natural language, and perception. The aim of AI is to create systems that can perform tasks autonomously or assist humans in tasks that require intelligence. Below are some of the key similarities between AI and ML.
1. Both Aim to Replicate Human Intelligence
The overall goal of both AI and ML is the replication of human intelligence and reasoning in machines. Although AI focuses on the broader goal of creating intelligent systems, ML is one of the basic methods AI systems use to learn and make decisions. In essence, both fields aim to enhance machines’ ability to perform tasks that require the thinking and cognitive abilities of humans.
Whether it’s AI systems powering autonomous vehicles or ML algorithms driving personalized recommendations, both technologies aim to simulate human intelligence in different degrees.
2. Dependence on Data to Function
Data is crucial to both AI and Machine Learning. AI systems, regardless of whether they incorporate ML, cannot function effectively without data. For instance, natural language processing (NLP) models require large amounts of text data to comprehend and generate human language. Similarly, vision systems on computer rely on labeled images to recognize objects, faces, or scenes.
The need for data in ML is even more important. Machine Learning models learn from data and continuously improve their accuracy based on the quality and quantity of the data. Both AI and ML leverage data to generate insights, make predictions, and enhance performance over time.
3. Automation of Tasks
Another major purpose of AI and ML is to automate tasks that previously required human abilities. AI-powered chatbots can handle customer inquiries, while ML algorithms can detect fraud in real time. Both technologies are impacting industries by automating complex processes.
For instance, popular AI-driven virtual assistants like Siri and Alexa use ML algorithms to understand voice commands and improve their responses over time. In essence, both AI and ML are designed to streamline workflows, reduce manual labor, and increase efficiency through automation.
4. Continuous Learning and Improvement
Both AI and ML systems are designed to evolve on their performance over time. AI can improve through various learning techniques, including Machine Learning, where algorithms are trained on new data. This continuous learning process helps AI systems become more effective in their various domains.
Machine Learning, in particular, is designed to learn and improve with experience. The more data it processes, the better it can recognize patterns, leading to more accurate predictions and decisions. This iterative learning process is central to both AI and ML, ensuring they evolve and improve their performance over time.
5. Broad Applications Across Multiple Industries
Both AI and ML have applications across various industries, from healthcare and finance to retail, entertainment, and transportation. In healthcare, AI and ML algorithms help diagnose diseases, predict patient outcomes, and recommend personalized treatments. In finance, they detect fraudulent transactions, assess credit risks, and automate trading strategies.
Their ability to analyze large datasets, automate processes, and make data-driven decisions has made both AI and ML extremely valued across numerous sectors. From voice recognition systems to self-driving cars, these technologies are evolving industries globally by enhancing efficiency and fostering innovation.
It is evident that although Artificial Intelligence and Machine Learning are not the same, they share similarities that highlight their interdependence and mutuality. Both aim to replicate human intelligence, rely on data, automate tasks, continuously improve, and have widespread applications across industries. Having explored their similarities, we will equally dive into their unique features. Check out our next post, where we will highlight the unique contributions of Machine Learning and Artificial Intelligence to the tech ecosystem.